The School of Atmospheric Sciences at Nanjing University, China, and the Earth System Numerical Prediction Center of the China Meteorological Administration have published a paper, Impacts of lateral boundary conditions from numerical models and data-driven networks on convective-scale ensemble forecasts, in Atmospheric and Oceanic Science Letters. which outlines the impacts of the lateral boundary conditions provided by the AI models ‘Pangu-weather’ and F’uxi-weather”‘ on convective-scale ensemble prediction systems.
The future of convective-scale ensemble forecasts
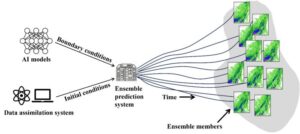
Overall, this work shows that AI weather models have the ability to replace traditional global numerical weather models in providing lateral boundary conditions for convective-scale ensemble prediction systems. Moreover, as the vertical resolution of AI weather models improves, it is expected that further positive impacts on convective-scale ensemble forecasts will materialize.
Evaluating the effectiveness of AI
The research set out to investigate the performance of using the predictions from the AI weather models as lateral boundary conditions to drive convective-scale ensemble prediction systems. This is because AI weather models can produce forecasts for the next seven to 10 days in just a few minutes, which is around 10,000 times faster than numerical weather models.
The team found that the results of the ensemble prediction system using lateral boundary conditions from Pangu-weather forecasts were found to be comparable to those using the conditions from National Centers for Environmental Prediction Global Forecast System forecasts.
Meanwhile, the results using lateral boundary conditions from Fuxi-weather forecasts showed slightly inferior performance compared to the others. When the number of vertical levels of the Global Forecast System forecasts was reduced from 33 to 13 (the same as Pangu-weather and Fuxi-weather), the results were the worst among all ensemble prediction systems. This indicates that the vertical resolution of the lateral boundary conditions could significantly affect the convective-scale ensemble prediction systems.
In related news, researchers from the Institute of Oceanology of the Chinese Academy of Sciences (IOCAS) have developed a new model for forecasting rapid intensification (RI) of a tropical cyclone (TC) based on ‘contrastive learning’. This study was published in the Proceedings of the National Academy of Sciences (PNAS). Click here to read the full story.